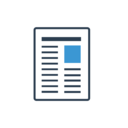
From heterogeneous healthcare data to disease-specific biomarker networks: A hierarchical Bayesian network approach.


In this work, we introduce an entirely data-driven and automated approach to reveal disease-associated biomarker and risk factor networks from heterogeneous and high-dimensional healthcare data. Our workflow is based on Bayesian networks, which are a popular tool for analyzing the interplay of biomarkers. Usually, data require extensive manual preprocessing and dimension reduction to allow for effective learning of Bayesian networks. For heterogeneous data, this preprocessing is hard to automatize and typically requires domain-specific prior knowledge. We here combine Bayesian network learning with hierarchical variable clustering in order to detect groups of similar features and learn interactions between them entirely automated. We present an optimization algorithm for the adaptive refinement of such group Bayesian networks to account for a specific target variable, like a disease. The combination of Bayesian networks, clustering, and refinement yields low-dimensional but disease-specific interaction networks. These networks provide easily interpretable, yet accurate models of biomarker interdependencies. We test our method extensively on simulated data, as well as on data from the Study of Health in Pomerania (SHIP-TREND), and demonstrate its effectiveness using non-alcoholic fatty liver disease and hypertension as examples. We show that the group network models outperform available biomarker scores, while at the same time, they provide an easily interpretable interaction network.
SEEK ID: https://seek.lisym.org/publications/309
PubMed ID: 33577591
Projects: LiSyM Pillar I: Early Metabolic Injury (LiSyM-EMI)
Publication type: Journal
Journal: PLoS Comput Biol
Citation: PLoS Comput Biol. 2021 Feb 12;17(2):e1008735. doi: 10.1371/journal.pcbi.1008735. eCollection 2021 Feb.
Date Published: 13th Feb 2021
Registered Mode: by PubMed ID

Views: 1633
Created: 28th Jun 2021 at 21:20
Last updated: 8th Mar 2024 at 07:44

This item has not yet been tagged.

None