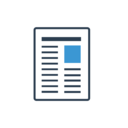
Model Order Reduction (MOR) of Function‐Perfusion‐Growth Simulation in the Human Fatty Liver via Artificial Neural Network (ANN)


Numerical modeling of biological systems has become an important assistance for understanding and predicting hepatic diseases like non‐alcoholic fatty liver disease (NAFLD) or the detoxification of drugs and toxines by the liver. We developed a model for the simulation of hepatic function‐perfusion processes using a multiscale and multiphase approach. Here, the liver lobules are described using a homogenization approach with a coupled set of partial differential equations (PDE) based on the Theory of Porous Media (TPM) to describe the coupled blood transport and tissue deformation. For the description of metabolic processes on cellular scale ordinary differential equations (ODE) are used. For many practical and clinical applications, e.g. optimization procedures or uncertainty quantification, a fast but reliable computation is required. Thus, we use a non‐linear model order reduction (MOR) based on an artificial neural network (ANN) for the prediction of simulation results. The practicability of this approach is shown in a comparison between the high fidelity numerical simulation of a NAFLD and the predicted results by the ANN.
SEEK ID: https://seek.lisym.org/publications/254
Projects: Multi-Scale Models for Personalized Liver Function Tests (LiSyM-MM-PLF)
Publication type: Journal
Journal: PAMM
Citation: Proc. Appl. Math. Mech. 19(1)
Date Published: 1st Nov 2019
Registered Mode: by DOI

Views: 1506
Created: 26th Jul 2020 at 10:11
Last updated: 8th Mar 2024 at 07:44

This item has not yet been tagged.

None